Self-Supervised Reversibility-Aware Reinforcement Learning (SRRL) is an advanced technique in the field of artificial intelligence and machine learning, particularly within reinforcement learning (RL). It aims to enhance the learning efficiency and robustness of RL agents by incorporating the concept of reversibility into the learning process. Here’s an in-depth look at SRRL:
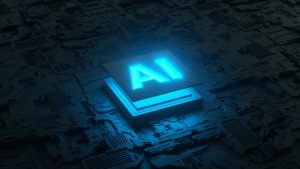
Reinforcement learning involves training agents to make sequential decisions by interacting with an environment to maximize cumulative rewards. Traditional RL methods often struggle with issues such as sparse rewards and exploration inefficiency. SRRL addresses these challenges by leveraging the idea that actions taken by an agent in an environment can often be reversed, thereby providing additional self-supervised signals to guide the learning process. Self-Supervised Reversibility-Aware Reinforcement Learning represents a significant advancement in the field of RL by introducing the concept of reversibility as a self-supervised signal. By leveraging reversible actions, SRRL enhances exploration efficiency, learning robustness, and the overall performance of RL agents. As research progresses, SRRL is poised to contribute substantially to the development of more intelligent and adaptable AI systems capable of thriving in complex and dynamic environments.